AI: Financial analysts will be replaced or augmented by LLMs, see $2.5B AlphaSense
The real promise of LLMs in financial analysis isn't just replicating what a human analyst can do, but uncovering insights that humans miss
Hi Fintech Futurists βΒ
Today we highlight the following:
AI: LLMs officially outperform financial analysts - can live audio and video analysis take them further?
LONG TAKE: What Apple's AI push means for Fintech and Privacy
PODCAST: What makes a successful AI investor, with Fusion Fund Founder Lu Zhang
CURATED UPDATES: Machine Models, AI Applications in Finance, Infrastructure & Middleware
To support this writing and access our full archive of newsletters, analyses, and guides to building in the Fintech & DeFi industries, see subscription options here.
In Partnership
Ground-breaking research from Cornerstone Advisors and TruStageTM uncovers the impact of embedded loan payment insurance on lenders:
Reduce defaults
Attract borrowers at a lower cost
Increase revenue
Attract more capacity
Differentiate offerings
AI: LLMs officially outperform financial analysts - can live audio and video analysis take them further?
Since ChatGPT went mainstream, there has been much debate about whether large language models (LLMs) can make informed financial decisions.
Most papers on GPT-based earnings and stock predictions lack a robust methodology β they overlook factors like statistical significance, proper accuracy metrics, and LLM flaws such as consistency and hallucination. This usually leads to overly positive results, causing many of these papers to be viewed with suspicion. Previous research about using neural networks, tree methods, or NaΓ―ve Bayes for earnings predictions employed much more rigorous methodologies.
Still, the commercial opportunity is there. For example, the market intelligence platform AlphaSense recently acquired Tegus, a provider of expert research and financial data, and raised $650MM in funding. AlphaSense is running at $200MM in revenue and has combined market intelligence and equity research into a powerful platform β a lifeline given the pressure on equity commission revenues. It has been experimenting with generative AI for financial statement analysis, offering its AlphaSense Large Language Model (ASLLM), which allows users to upload and manage millions of documents and perform tasks like sentiment analysis, topic extraction, and smart summaries.
If data is the oil from which machine intelligence is summoned, repositories of best-in-class analysis like AlphaSense β as well as the large investment banks, the rating agencies (e.g., Moodyβs), and the data aggregators (e.g., Morningstar) β have an advantage in building out a financial analyst mind.
In our previous AI analysis, we touched upon the paper, "Can ChatGPT Forecast Stock Price Movements? Return Predictability and Large Language Models," but it left many questions about the methods used. Now, researchers from the University of Chicago have published a more rigorous and believable paper titled "Financial Statement Analysis with Large Language Models."Β
The paper, which has already garnered 50,000 downloads, explores whether an LLM can perform financial statement analysis like professional human analysts. The answer isn't straightforward, as an LLM lacks the contextual understanding of a company's financials. Further, working with numbers is particularly challenging for language models.
To test an LLM's performance, the researchers anonymized and standardized balance sheets and income statements, removing company names and replacing years with labels such as t and tβ1. They then used Chain-of-Thought (CoT) prompts to guide the model through financial statement analysis and predict future earnings. The model's performance was evaluated using data from the Compustat and IBES databases, covering the period from 1968 to 2021, with 150,678 firm-year observations from 15,401 distinct firms. The analyst sample spans from 1983 to 2021, with 39,533 observations from 3,152 firms.
The target variable, which is what the model aims to predict, was the directional change in future earnings. Analysts' prediction accuracy was measured using consensus forecasts to ensure comparability with the model's predictions.
The researchers used two evaluation metrics: accuracy and F1-score. Accuracy is the percentage of correctly predicted cases, while the F1-score combines precision and recall (the harmonic mean) into a single measure. Analysts' predictions for one-year-ahead earnings have 52.71% accuracy and a 54.48% F1-score, which is better than a naive model with 49.11% accuracy and a 53.02% F1-score. Analysts' accuracy improves to 55.95% for month-three and 56.58% for month-six forecasts.
GPT, using a simple prompt, achieves similar results with 52.33% accuracy and a 54.52% F1-score. However, when using chain-of-thought (CoT) reasoning, GPT's accuracy increases to 60.35%, significantly outperforming analysts' one-month predictions. This improvement is statistically significant at the 1% level. Notably, the language model only used balance sheet and income statement data, without any additional narrative or context.
To us, the most exciting aspect of the paper is the asset pricing tests, which assess the practical value of the analysis through trading strategies based on GPT's predictions. According to Fama and French, signals indicating future expected profits should correlate positively with expected stock returns. If GPT forecasts contain additional information about future profitability, they should also predict future stock returns. So the researchers formed investment strategies using GPT's predictions of earnings direction and compared these with strategies based on ANN and logistic regression forecasts.
The approach involves forming portfolios on June 30 each year, holding them for one year, and measuring their Sharpe ratios and monthly alphas. Stocks are sorted into portfolios based on GPT's binary directional predictions, the predicted magnitude of earnings changes, and log probability values. Specifically, for each fiscal year, stocks predicted to experience an βincreaseβ in earnings with a predicted magnitude of either βmoderateβ or βlargeβ are selected. These stocks are then sorted by their average log probability values, retaining those with the highest log probabilities to form a decile portfolio. The same method is applied to stocks predicted to experience a βdecreaseβ in earnings, creating a corresponding short portfolio.
The results show that equal-weighted portfolios based on GPT predictions achieve a Sharpe ratio of 3.36, outperforming those based on ANN (2.54) and logistic regression (2.05). For value-weighted portfolios, ANN performs better (Sharpe = 1.79) than GPT (1.47), but both surpass logistic regressions (0.81). Monthly alpha calculations reveal that GPT-based equal-weighted portfolios generate a monthly alpha of 84 basis points (10% annually), even after controlling for multiple factors, outperforming ANN and logistic regression portfolios. And long-short portfolios based on GPT predictions consistently outperform the market portfolio, even during market downturns.Β
In our last AI analysis, we discussed how Google doubled Geminiβs context window to 2 million tokens and improved audio and image understanding. As LLMs become better at analyzing different types of data, their ability to forecast earnings and stock prices could greatly improve.
During the pandemic, there was a surge of research using computer vision for financial predictions. New tools were developed to analyze FOMC press conferences, capturing details like facial expressions, discussion complexity, how often the Chair looks down to read documents, posture, and word count. Researchers created datasets of video images from these press conferences and used deep learning algorithms to extract nonverbal communication data that couldn't be gathered from written transcripts.
For example, in the paper, βRisk & Returns around FOMC Press Conferences: A Novel Perspective from Computer Visionβ, Alexis Marchal found that complex discussions were linked to higher equity returns and lower volatility.Β
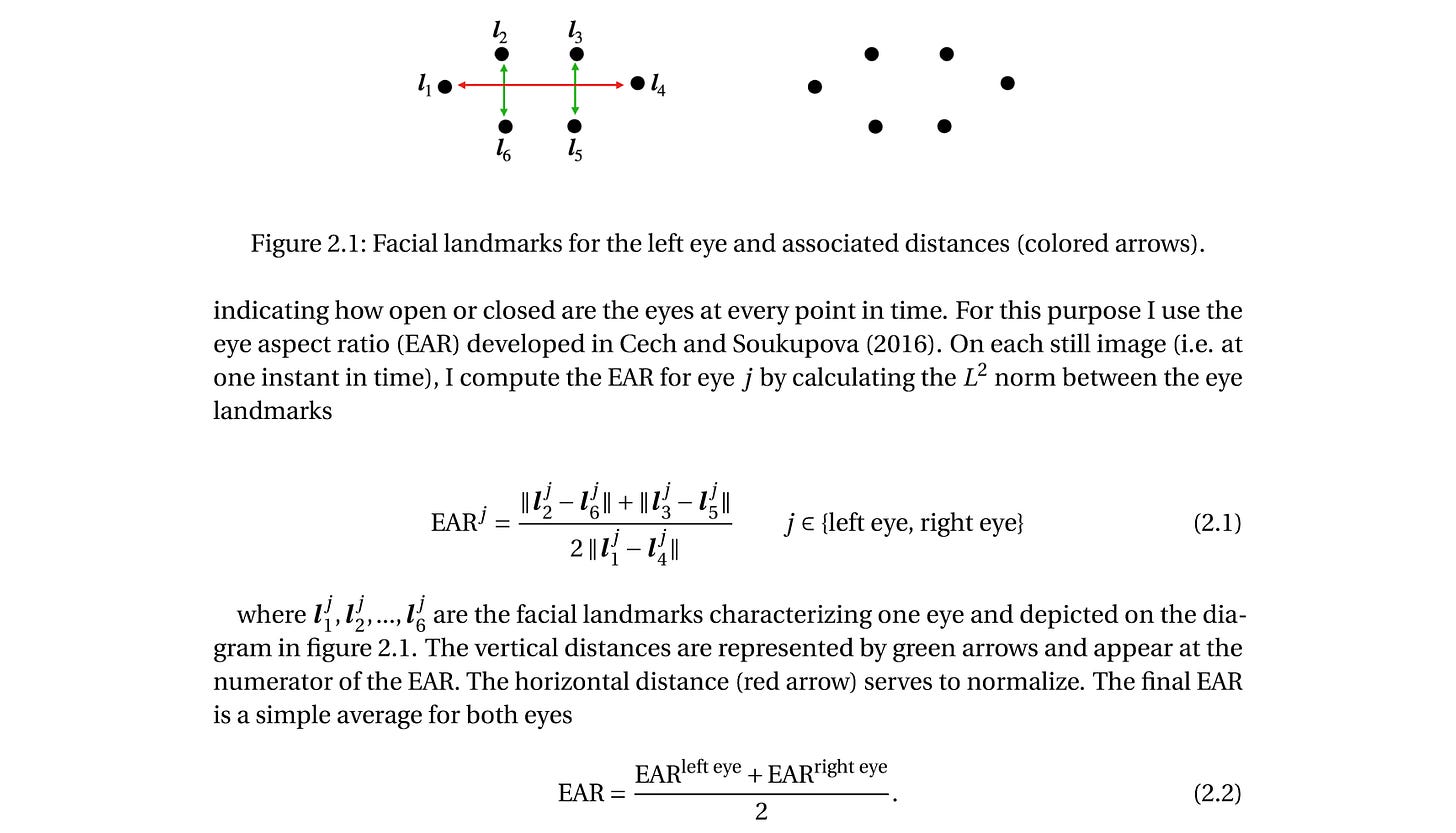
We are going to see the immense firepower of artificial intelligence trained squarely on all media related to the economy. With the advancement of multimodal LLMs, AI agents will participate in real-time earnings calls and press conferences to see if these models can capture market sentiment from live events.
The real promise of LLMs in financial analysis isn't just replicating what a human analyst can do β they will do this at a minimum. Rather, the potential is to uncover insights that we mortals miss with a speed and precision yet to be imagined.
π Related Coverage π
Blueprint Deep Dive
Long Take: What Apple's AI push means for Fintech and Privacy (link here)
In this article, we discuss the competitive landscape among Microsoft, Apple, and NVIDIA, all vying for the title of the world's most valuable company, with a combined market cap of $10 trillion. The driving force behind their growth is generative AI and foundation models. Apple recently launched its AI initiative, Apple Intelligence, with features integrated into the operating system, enhancing tools like Siri.
This move positions Apple to dominate AI just as it did with previous technologies, potentially sidelining smaller competitors. However, this raises concerns about privacy and the broader implications of AI integration into daily life.
ποΈ Podcast Conversation: What makes a successful AI investor, with Fusion Fund Founder Lu Zhang (link here)
In this conversation, we chat with Lu Zhang - Founder and Managing Partner of Fusion Fund, is a renowned Silicon Valley-based investor, a serial entrepreneur, and a Stanford Engineering alumna. With a strong technical background, Lu has extensive experience bringing a broad range of technologies to commercialization and deep domain expertise in AI in healthcare, Enterprise AI/Networks, Edge Computing, and Data Privacy.
Prior to starting Fusion Fund, Lu was a serial entrepreneur and materials science researcher. Lu is a first-generation immigrant originally from Inner Mongolia. At the age of 21, she built a medical device company for Type II Diabetes diagnosis based on her graduate school research in Stanford University. Following the acquisition of her startup by a leading public medical device company, Lu began investing in and supporting early-stage entrepreneurs. This eventually led her to create Fusion Fund in 2015. Since then, Lu has built a distinguished eco system and established her reputation in the VC industry. She was honored as Young Global Leader by World Economic Forum (Davos), Silicon Valley Women of Influence, Best 25 Female early-stage investor by Business Insider, Featured Honoree of VC of Forbes 30 under 30 and Town & Country 50 Modern Swans - Entrepreneurship Influencer.
Curated Updates
Here are the rest of the updates hitting our radar.
Machine Models
β Theoretical Foundations of Deep Selective State-Space Models - Nicola Muca Cirone, Antonio Orvieto, Benjamin Walker, Cristopher Salvi, Terry Lyons
β Variational Bayesian Last Layers - James Harrison, John Willes, Jasper Snoek
Leave No Context Behind: Efficient Infinite Context Transformers with Infini-attention - Tsendsuren Munkhdalai, Manaal Faruqui, Siddharth Gopal
Universal Randomised Signatures For Generative Time Series Modelling - Francesca Biagini, Lukas Gonon, Niklas Walter
Misam: Using ML In Dataflow Selection of Sparse-Sparse Matrix Multiplication - Sanjali Yadav, Bahar Asgari
Automated Design of Linear Bounding Functions for Sigmoidal Nonlinearities in Neural Networks - Matthias KΓΆnig, Xiyue Zhang, Holger H. Hoos, Marta Kwiatkowska, Jan N. van Rijn
AI Applications in Finance
β Financial Statement Analysis with Large Language Models - University of Chicago Booth School of Business
β Detecting Multivariate Market Regimes Via Clustering Algorithms - James Mc Greevy, Aitor Muguruza, Zach Issa, Cristopher Salvi, Jonathan Chan, Zan Zuric
Multi-Factor Model with Time-Varying Volatility: A Multi-Task Learning Approach - Seoul National University
Reinforcement Learning for Jump-Diffusions - Xuefeng Gao, Lingfei Li, Xun Yu Zhou
Dynamic Knowledge Graph Asset Pricing - Victor Xiaohui Li, Yixiao Tan
A First Look at Financial Data Analysis Using ChatGPT-4o - Zifeng Feng, Bingxin Li, Feng Liu
Infrastructure & Middleware
β OpenAI Selects Oracle Cloud Infrastructure to Extend Microsoft Azure AI Platform - Financial Times
β Microsoft Will Spend $3.2B On Swedish AI Infrastructure - PYMNTS
Tether To Invest $1B In AI, Biotech, And Financial Infrastructure - Crypto Daily
Vertiv Introduces AI Power And Cooling Solutions For Data Centre Infrastructure In EMEA - Techerati
Snowflake's Platform Capabilities Accelerate AI Adoption in the Middle East - IDC
π Postscript
Sponsor the Fintech Blueprint and reach over 200,000 professionals.
π Reach out here.Read our Disclaimer here β this newsletter does not provide investment advice
For access to all our premium content and archives, consider supporting us with a subscription. In addition to receiving our free newsletters, you will get access to all Long Takes with a deep, comprehensive analysis of Fintech, Web3, and AI topics, and our archive of in-depth write-ups covering the hottest fintech and DeFi companies.
"Understanding financial reports is like having a crystal ball to see into the business world, just like Buffett and Munger." Although this statement may sound exaggerated, it also indirectly confirms the importance of the ability to interpret financial statements.
With the rapid development of AI, this professional gap has been quickly bridged. Generative artificial intelligence, represented by ChatGPT, has already achieved professional-level financial analysis capabilities.