AI: What OpenAI can learn from the world's largest quant hedge fund
The 'Renaissance Paradox' and the future of AI training
Hi Fintech Futurists —
Today we highlight the following:
AI: The 'Renaissance Paradox' and the future of AI training
LONG TAKE: Understanding the $1B MoneyLion acquisition by Gen Digital
PODCAST: Understanding Decentralized AI Networks with 0G Labs CEO, Michael Heinrich
CURATED UPDATES: Machine Models, AI Applications in Finance, Infrastructure & Middleware
To support this writing and access our full archive of newsletters, analyses, and guides to building in the Fintech & DeFi industries, see subscription options here.
AI: The 'Renaissance Paradox' and the future of AI training
In our last AI analysis, we noted that training LLMs is hitting a wall — and increasing investment in compute power may no longer be the solution. Costs have surged from $1 million in 2021 to $100 million in 2024, with future models projected to cost between $1 billion and $10 billion. Despite these escalating costs, the ROI for training by new entrants remains uncertain.
At the recent NeurIPS conference, Ilya Sutskever, OpenAI’s co-founder and former Chief Scientist, warned, “Pre-training as we know it will unquestionably end.” He likened the situation to the finite nature of fossil fuels, noting that the internet’s supply of human-generated content — essential for training LLMs — is limited. According to Sutskever, this constraint will force a shift in training methods, pushing the industry toward agentic systems capable of interacting with the real world.
“We have to deal with the data that we have. There’s only one Internet.” - Ilya Sutskever
Gary Marcus, NYU Professor Emeritus and a long-time AI skeptic, has been raising similar concerns for years. He argues, “there is no principled solution to hallucinations in systems that traffic only in the statistics of language without explicit representation of facts and explicit tools to reason over those facts.” The soaring cost of training exacerbates the problem. If no company secures a unique edge, LLMs risk becoming commodities, which could trigger price competition, squeeze revenues, and potentially impact the valuations of tech giants like NVIDIA.
This divide in the AI community is apparent. Critics question whether current methods will ever lead to superintelligent AI, citing the lack of true reasoning and the unpredictability of these models. Meanwhile, optimists believe that AI-generated data, self-evaluation and agents offer a path forward.
The industry is encountering what could be called a 'Renaissance Paradox' - named after Renaissance Technologies, the quant fund that capped its Medallion fund after discovering that increased scale led to deteriorating returns. Instead of pursuing size, Renaissance focused on extracting better signals from existing data.
In a similar vein, the AI industry is discovering that simply scaling up compute power produces diminishing returns. While Renaissance chose to cap its Medallion fund, the AI industry has no such luxury of choice; it has hit a hard constraint. The challenge now is finding more efficient ways to use the data we already have.
As we hinted above, the dilemma is familiar to the finance industry. For decades, financial models relied on very finite (relatively speaking) traditional time-series data — historical market returns and economic indicators — rooted in econometric techniques. Strategies like momentum, quality, and value investing emerged from this tradition. However, the 2007 quant crisis exposed the limitations of these methods. The widespread use of similar datasets, models, and trading strategies led to a dangerous lack of diversification, amplifying market risks and resulting in collective failures when market anomalies occurred.
In response, the finance industry turned to alternative data — sources like satellite imagery, social media sentiment, and transaction records — to gain new insights. While valuable, alternative data is often unstructured, computationally intensive, and expensive to process. But once again, there’s a risk of commoditization: when too many firms access the same datasets, the competitive edge erodes. With most quant firms now having access to similar data vendors, infrastructure, and machine learning models, differentiation is increasingly difficult.
This has led to the rise of synthetic data generation. By creating realistic, privacy-compliant datasets, institutions can simulate market scenarios and test machine learning models without being constrained by finite real-world data. Quant funds have been leveraging synthetic data for years to extract alpha. See here and here for some examples. A non-finance case study is Tesla, which relied on massive real-world driving datasets to develop self-driving technology. Yet by 2022, even after billions of miles of data collection, full autonomy remained elusive. In contrast, Waymo pivoted to synthetic data and simulation, generating variations of rare edge cases that real-world data couldn’t provide.
The same principles are now playing out in AI.
“Getting the balance right between synthetic and real-world data will determine the future of AI—its capabilities, limitations and, ultimately, its impact on society.” - IBM researcher Akash Srivastava
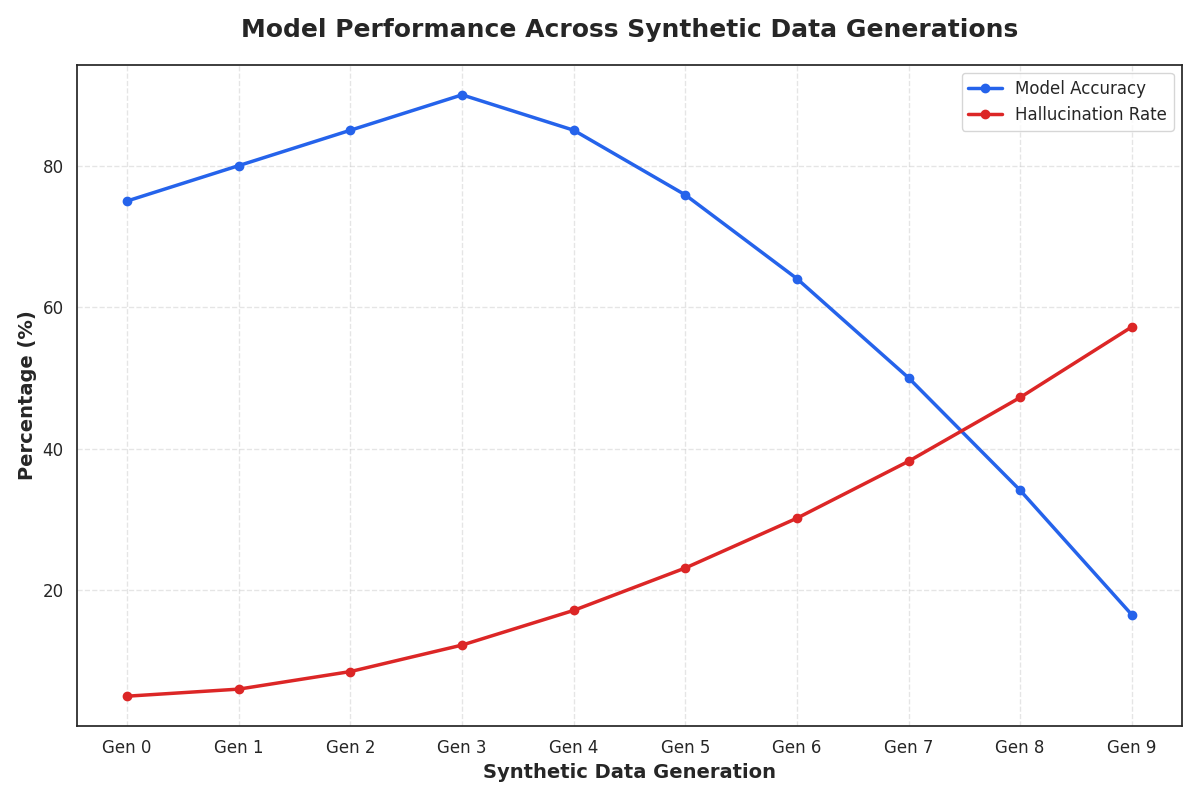
Looking ahead, the winners in the AI industry won’t necessarily be those with the largest datasets or the most compute power (although they will have to possess both). Success will come from those who can squeeze the most out of their data. In other words, advances in data efficiency — not data scale — will drive the next breakthroughs.
We’re already seeing this shift with AI agents, which actively learn through interaction with their environment rather than relying solely on passive training from pre-collected datasets. These agents can generate their own experiences, simulate scenarios, test hypotheses, and improve their performance through iterative trial and error.
However, this shift toward synthetic data and agent-based learning isn't without risk. If models become too reliant on synthetic data, trained on multiple generations of machine-generated information, we could see a situation where small imperfections in early generations compound into significant distortions over time.
👑Related Coverage👑
Blueprint Deep Dive
Analysis: Understanding the $1B MoneyLion acquisition by Gen Digital (link here)
The neobank landscape has evolved dramatically since the SPAC boom and subsequent crash, with many fintech companies like MoneyLion enduring public market turmoil but adapting successfully.
MoneyLion, originally valued at $3B during its SPAC debut, is being acquired by Gen Digital for $1B, highlighting how strategic buyers are leveraging underpriced fintech assets. Gen Digital, known for cybersecurity products like Norton and Avast, aims to integrate MoneyLion into its "financial safety" offerings, though the strategic fit appears more opportunistic than intuitive. We discuss the economics and rationale of the acquisition.
🎙️ Podcast Conversation: Understanding Decentralized AI Networks with 0G Labs CEO, Michael Heinrich (link here)
Lex interviews Michael Heinrich, co-founder and CEO of 0G (Zero Gravity) Labs, a decentralized AI network that has recently raised $290MM at around a $2B valuation.
In this episode, Lex and Michael explore the concept of decentralized artificial intelligence (AI) and how it differs from centralized, closed-source AI models provided by big tech companies. Michael kicks off with his background in tech and finance, and how he became interested in decentralized AI as a solution to the issues with closed AI systems.
Curated Updates
Here are the rest of the updates hitting our radar.
Machine Models
Understanding Transfer Learning via Mean-field Analysis - Gholamali Aminian, Łukasz Szpruch, Samuel N. Cohen
Scalable Signature-Based Distribution Regression via Reference Sets - Andrew Alden, Carmine Ventre, Blanka Horvath
Strategic Collusion of LLM Agents: Market Division in Multi-Commodity Competitions - Caltech
Unsupervised-to-Online Reinforcement Learning - KAIST, UC Berkeley
When to Sense and Control? A Time-adaptive Approach for Continuous-Time RL - ETH Zurich
Mining Causality: AI-Assisted Search for Instrumental Variables - Sukjin Han
AI Applications in Finance
⭐ AI and Finance - UCLA
⭐ Deep Learning Interpretability for Rough Volatility - University of Cambridge, Imperial College London
Design choices, machine learning, and the cross-section of stock returns - Technical University of Munich
Assessing the Performance of AI-Labelled Portfolios - University of Innsbruck
Dynamic Graph Representation with Contrastive Learning for Financial Market Prediction: Integrating Temporal Evolution and Static Relations - University of Bristol
Unsupervised learning-based calibration scheme for Rough Bergomi model - Changqing Teng, Guanglian Li
(Deep) Learning Analyst Memory - University of Chicago
Infrastructure & Middleware
European AI infra company Nebius nabs $700MM from Nvidia, Accel, others - TechCrunch
Crusoe secures $600MM for AI infrastructure expansion - Verdict
Meta signs deal with Oracle Cloud for AI training - DCD
Jensen Huang Wants to Make AI the New World Infrastructure - Wired
🚀 Postscript
Sponsor the Fintech Blueprint and reach over 200,000 professionals.
👉 Reach out here.Check out our new AI products newsletter, Future Blueprint. (Don’t tell anyone)
Read our Disclaimer here — this newsletter does not provide investment advice
For access to all our premium content and archives, consider supporting us with a subscription. In addition to receiving our free newsletters, you will get access to all Long Takes with a deep, comprehensive analysis of Fintech, Web3, and AI topics, and our archive of in-depth write-ups covering the hottest fintech and DeFi companies.