AI: Is Goldman wrong about Big Tech overspending $1 Trillion on AI infra?
When does that investment get paid back in revenue?
Hi Fintech Futurists โย
Today we highlight the following:
AI: Will the $1 Trillion AI infrastructure bet meet revenue expectations?
LONG TAKE: Monetization of financial data at $4B Envestnet, $170B Intuit, and $7B SoFi
PODCAST: Embedding Insurance in digital lending, with Danielle Sesko of TruStage
CURATED UPDATES: Machine Models, AI Applications in Finance, Infrastructure & Middleware
To support this writing and access our full archive of newsletters, analyses, and guides to building in the Fintech & DeFi industries, see subscription options here.
AI: Will the $1 Trillion AI infrastructure bet meet revenue expectations?
AI stocks are on a remarkable rise, with global AI spending surpassing nearly all predictions made before the generative AI boom.
By mid-2024, AI infrastructure investments have continued to soar, prompting some important questions โ How much value does AI need to create to justify these valuations? Is there enough demand for all this infrastructure? And what is at stake if this is a bubble that bursts?
Goldman Sachs has released a research report featuring the views of Jim Covello, Head of Global Equity Research, among others industry contributors, that suggests we might be in the midst of another bubble. It questions whether the expected $1 trillion investment in AI over the next few years will face the same fate as the billions poured into ventures like VR and the metaverse.
Consider that investments into early Internet telecoms faced similar questions, and ended up over-building capacity in the early days.
Goldman warns that the market might be too optimistic about AI โ "Today's hyperscalers will eventually need to prove that their investments will generate revenues and earnings." That means applications need to make money.
Currently, there is still a large gap between the revenue expectations driven by the AI infrastructure boom and the actual revenue growth in the AI sector. That means it may be difficult to get a tangible return on investments. We explore below whether this leads to a painful correction sometime soon, as Goldman Sachs predicts, or whether we will derive sufficient value from these infrastructure developments.
Letโs look at recent AI-related investments by the main โhyperscalersโ โ think Big Tech with billions of users.
Sequoia Capital predicts that 2025 will be the โYear of the Data Center,โ marking a shift from a hype cycle to an industrial-driven build cycle. Goldman Sachs's report also highlights a phase of AI investment that centers on AI infrastructure, encompassing semiconductor firms, cloud providers, data center REITs, hardware and equipment companies, security software stocks, and utility companies.
Here are some key points we quote directly from Sequoiaโs analysis:
Amazon โ $50B in new projects, including 216 new buildings. Over the next 15 years, the company is planning on spending between $100B and $150B over the next 15 years. This includes $11B campus in Indiana; $10B across two campuses in Mississippi; $5.3B in new data centers in Saudi Arabia; a nuclear-powered data center near Pennsylvania; a new planned data center in Texas; and a $15B commitment in Japan.
Microsoft โ expanded its energy capacity to 5GW and is doubling new data center construction in 2024. Projects include $3.3B in Wisconsin; $1B in Indiana; $1B in Georgia; $4.3B in France; $3.5B in Germany; $3.2B in the UK; $3.2B in Sweden; $2.1B in Spain; $2.2B in Malaysia; $1.7B in Indonesia; $1B in Kenya, and a new data center in Mexico.
Google โ a $2B data center in Indiana; a $1B data center in Kansas City, Missouri; a $1.1B data center in Finland; $576MM data center in Iowa
Meta โ recently announced it has accumulated 350,000 H100 GPUs, as part of a total fleet of 600,000 H100 equivalents, and two 24,000 GPU clusters dedicated to Llama 3 training. New data centers are planned in Idaho; Texas; Iowa; and Wyoming.
As you can, investment to scale up AI is tremendous and has been reflected by enthusiasm in the stock market. It is also starting to impact overall energy demand for the entire United States as shown in the chart above.
Goldmansโ Covello tries to draw a sharp contrast between AI and past tech transitions. Traditionally, new tech has replaced expensive existing solutions with more affordable alternatives, disrupting incumbents through the innovatorโs dilemma.
The early days of the internet allowed e-commerce to flourish without the overhead of physical stores. In contrast, Goldman argues, AI development costs are significantly higher thereby making AI services uneconomical. However, we believe this is a short-term phenomenon โ upfront fixed capital investment, rather than ongoing variable costs. Sequoia predicts that once the new data center capacity comes online, the cost of training and inference services provided by AWS, Azure, and GCP will sharply drop.
Goldman also challenges the view that AI costs will decrease over time, pointing out that the dominance of key players like Nvidia in the GPU market limits the competitive pressure that typically drives down prices. It could be true that without significant competition, AI might remain a costly investment for many businesses subject to monopoly โ even with Nvidiaโs Blackwell GPUs are on the way, promising 2.5x better performance at only 25% more cost.
On the other hand, the GPU supply shortage seen in 2023 has eased, and it is now relatively easy to acquire GPUs with reasonable lead times.
Companies are feeling the pressure to invest in AI due to the hype and fear of missing out. This arms race mirrors past tech hype cycles where substantial investments were made in tech like VR and the metaverse โ many of which have yet to demonstrate significant real-world applications. In a recent McKinseyโs State of AI report, only 5% of respondents (46 out of 876) reported that a meaningful share of their organizationsโ EBIT was attributed to their deployment of gen AI. But businesses across industries are being pushed to adopt AI strategies despite uncertain returns.
Salesforce, for example, saw a notable drop in its stock price after substantial AI investments failed to boost revenue as expected. However, there is fear that SaaS companies will be negatively impacted by AI competition. As a result, Goldman suggests focusing on the companies that will benefit most from the AI infrastructure boom โ chip manufacturers, utilities, and cloud service providers.
Goldmanโs view does highlight some of the rapid tech advancements over the past year and the significant investments by hyperscalers, including tens of billions on AI tools. But the key to any tech cycleโs success is identifying a โkiller applicationโ โ something that generative AI is still exploring.
Other financial firms and investors, like Coatue, have a more optimistic view of the industry, as you can see below. Unlike the amorphous commercial returns from self-driving cars or quantum computing, AI is already useful and generating some value. The question isnโt whether it is commercially viable, but rather the speed and extent to which it will grow.
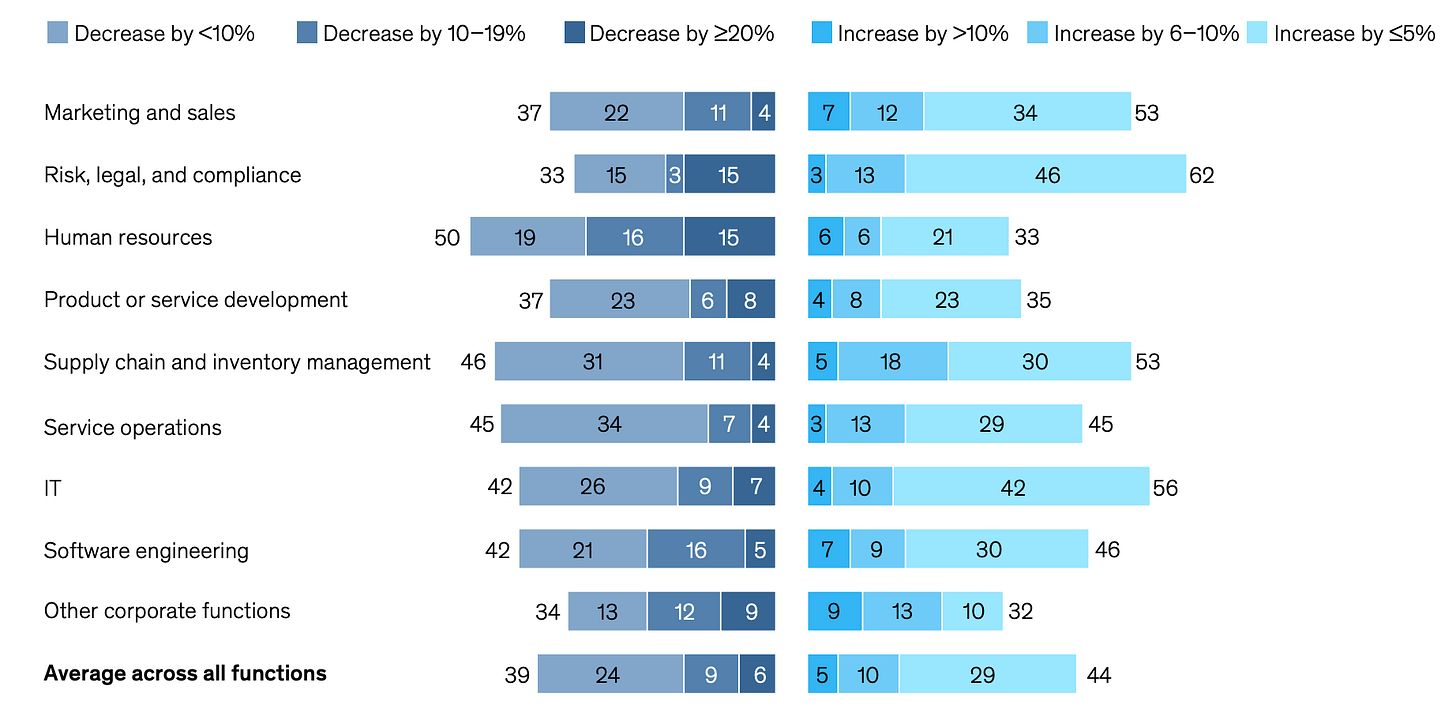
Wall Street expects $500B of revenue by the end of 2025 from the AI infrastructure sector. But we are not yet in bubble territory. The P/E ratio of the largest 10 tech stocks currently (31x) is still lower than during the peak of the Tech Bubble (52x) or late 2021 (43x).
The early winners could certainly be the component makers in the industrial supply chain and energy companies (e.g., electric utility). Investors pouring millions into near-identical AI image generators might not fare as well. Big tech companies like Apple and Meta will continue to deploy capital from their balance sheets. But longer term, we are deeply optimistic that the coming AI industrial phase will spark significant economic growth, which we call the machine economy.
๐ Related Coverage ๐
Blueprint Deep Dive
Long Take: Monetization of financial data at $4B Envestnet, $170B Intuit, and $7B SoFi (link here)
We examine Envestnet's potential sale to Bain Capital for $3.5 billion, with a detailed review of the economics of Envestnet, Intuit, and SoFi. Despite significant assets, Envestnet's relatively low valuation prompts questions about its unmet potential.
We compare Intuit's recent layoffs and SoFi's acquisition strategy, emphasizing the monetization challenges and declining importance of data aggregation. The analysis highlights how different approaches to leveraging financial data impact growth and profitability, suggesting that touching the consumer directly, as Intuit and SoFi do, proves more effective than purely B2B models.
๐๏ธ Podcast Conversation: Embedding Insurance in digital lending, with Danielle Sesko of TruStage (link here)
In this conversation, we chat with Danielle Sesko - Director of Product Management at TruStage. TruStage provides insurance coverage for loans in the digital lending space.
Danielle has been with TruStage for over 10 years and has held a variety of roles ranging from financial leadership to transformation and product development. Prior to joining TruStage Danielle spent her career in financial services and Mergers and Acquisitions. Danielle currently leads TruStageโs Digital Lending Insurance initiative which is focused on creating new digitally native products for new markets.
Curated Updates
Here are the rest of the updates hitting our radar.
Machine Models
โญ Gradient Boosting Reinforcement Learning - Nvidia
โญ Understanding Transformers via N-Gram Statistics - Google DeepMind
Your Transformer is Secretly Linear - HSE University, Lomonosov Moscow State University
Estimation of Spatio-temporal Extremes via Generative Neural Networks - Christopher Bรผlte, Lisa Leimenstoll, Melanie Schienle
Randomization Inference: Theory and Applications - David M. Ritzwoller, Joseph P. Romano, Azeem M. Shaikh
Learning Conditional Distributions on Continuous Spaces - Cyril Bรฉnรฉzet, Ziteng Cheng, Sebastian Jaimungal
How Neural Networks Learn the Support is an Implicit Regularization Effect of SGD - Pierfrancesco Beneventano, Andrea Pinto, Tomaso Poggio
Mitigating Catastrophic Forgetting in Language Transfer via Model Merging - Anton Alexandrov, Veselin Raychev, Mark Niklas Mรผller, Ce Zhang, Martin Vechev, Kristina Toutanova
Gaussian Process Interpolation with Conformal Prediction: Methods and Comparative Analysis - Aurรฉlien Pion, Emmanuel Vazquez
AI Applications in Finance
โญ GraphCNNpred: A stock market indices prediction using a Graph based deep learning system - California Institute of Technology
โญ Can AI with High Reasoning Ability Replicate Human-like Decision Making in Economic Experiments? - The University of Tokyo
Universal Randomised Signatures for Generative Time Series Modelling - Francesca Biagini, Lukas Gonon, Niklas Walter
Reinforcement Learning for Corporate Bond Trading: A Sell Side Perspective - Samuel Atkins, Ali Fathi, Sammy Assefa
MacroHFT: Memory Augmented Context-aware Reinforcement Learning On High Frequency Trading - Chuqiao Zong, Chaojie Wang, Molei Qin, Lei Feng, Xinrun Wang, Bo An
Infrastructure & Middleware
โญ The Physics of Learning: From Autoencoders to Truly Autonomous Learning Machines - Alex Ushveridze
The Quantum Imitation Game: Reverse Engineering of Quantum Machine Learning Models - Pennsylvania State University
Analyzing Machine Learning Performance in a Hybrid Quantum Computing and HPC Environment - Texas A&M University
OPIMA: Optical Processing-In-Memory for Convolutional Neural Network Acceleration - Colorado State University
Towards Efficient Deployment of Hybrid SNNs on Neuromorphic and Edge AI Hardware - University of South Carolina
๐ Postscript
Sponsor the Fintech Blueprint and reach over 200,000 professionals.
๐ Reach out here.Read our Disclaimer here โ this newsletter does not provide investment advice
For access to all our premium content and archives, consider supporting us with a subscription. In addition to receiving our free newsletters, you will get access to all Long Takes with a deep, comprehensive analysis of Fintech, Web3, and AI topics, and our archive of in-depth write-ups covering the hottest fintech and DeFi companies.